An updated (and condensed) version of our PQL 101 guide you can share with your team!
Defining and Identifying Product-Qualified Leads
Before we get into the main drivers of PQLs, I think it’s important we have a standard definition of what a Product Qualified Lead is. If we can all agree on this, then everything else will follow.
At Pocus we’ve been pushing for this universal definition: A Product-Qualified Lead (PQL) is a user or account that has shown strong product usage, fits your ideal customer profile (ICP), and/or has indicated buying intent.
You will have multiple PQL scores based on what playbooks (expansion vs. free trial conversion) your team is running.
Here’s a helpful framework you can use to start defining PQLs. Ask yourself these questions, write down answers and then go validate it with your data team.
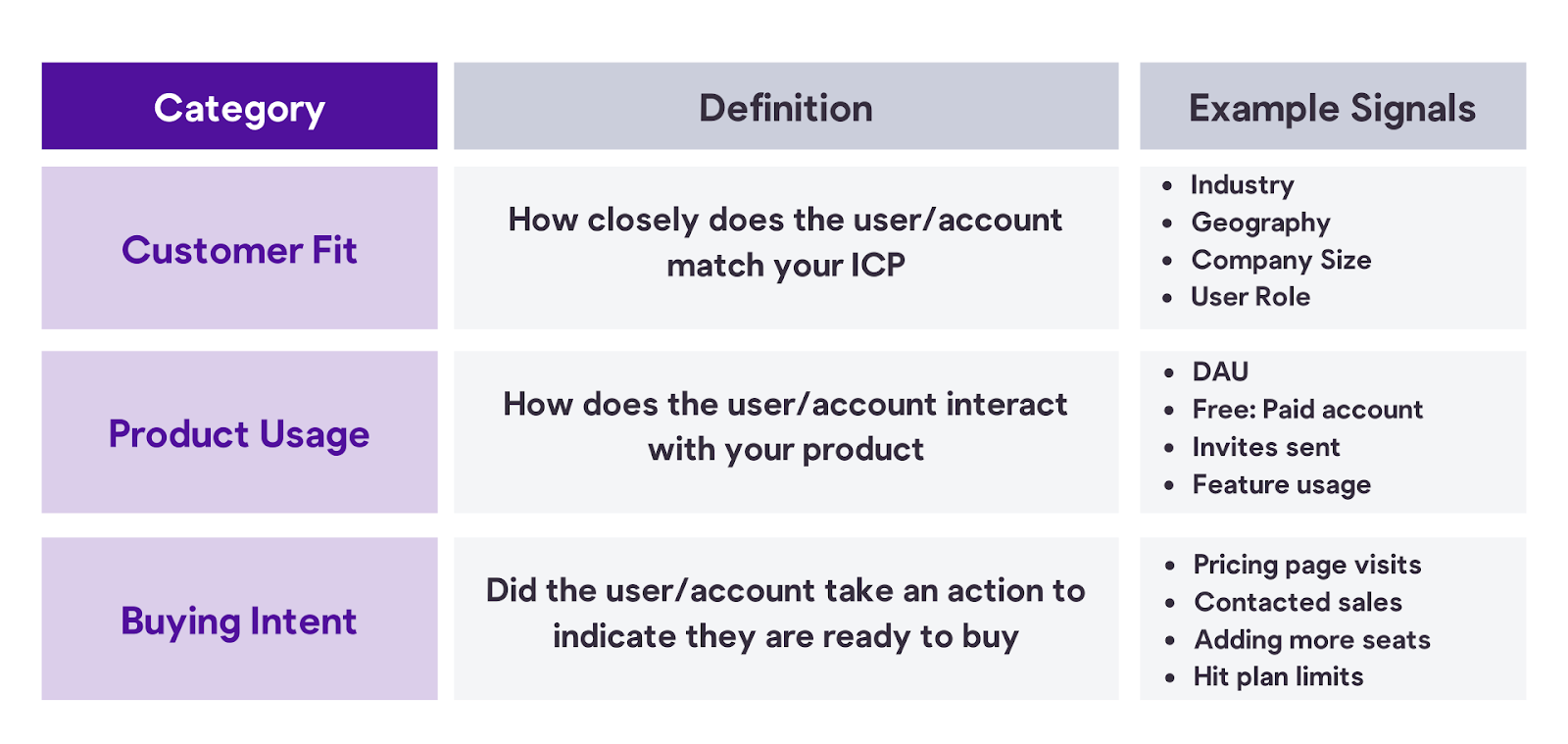
Although a PQL usually isn’t one single score, if you're just getting started it's ok to just have one because you are probably only focusing on a single playbook or goal.
Measuring fit, usage, and intent
- Customer fit: how closely the account and user matches your Ideal Customer Profile (ICP)(eg. industry, geography, company size, user role/title)
- Product usage: account and user level behavior (eg. DAU, frequency, recency, time spent, free:paid ratio, feature usage, virality, invites sent)
- Buying intent: buying intent (visited pricing page, clicked "talk to sales", new seats added to account)
These categories help you decide how to identify and prioritize various scenarios of leads. You’ll ideally look for customers with great customer fit and high product usage. But, you should also be aware of customers with great fit and low product usage, and vice-versa so that you can nurture those users or accounts to ensure they are realizing value from the product.
We find this matrix below helpful in understanding the various scenarios and mapping your PQL definition (hint: it’s up and to the right).
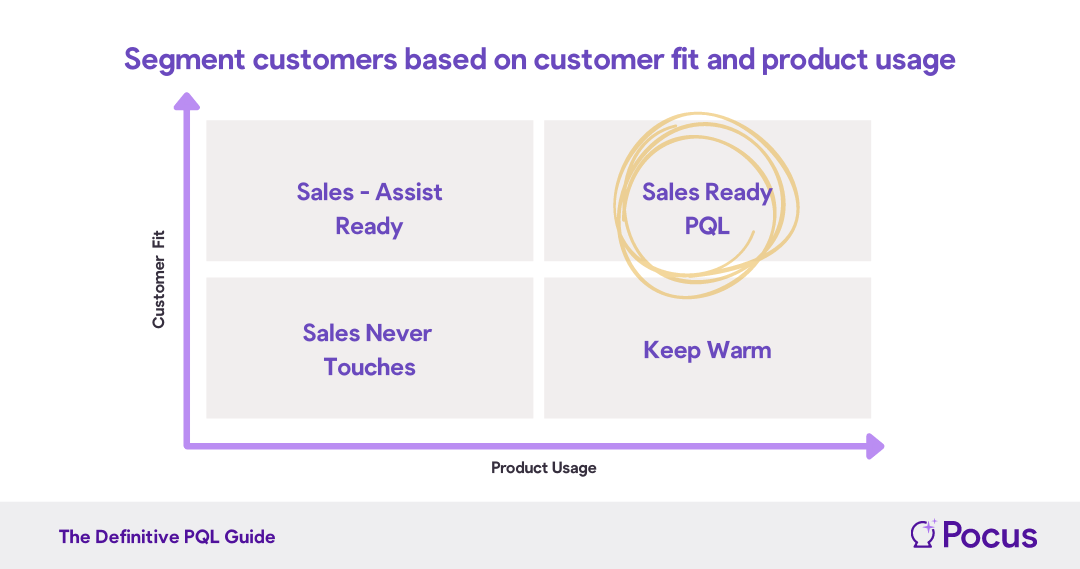
Each of the 4 segmentation scenarios require unique next steps from your sales team
- Sales Ready PQLs: these are your PQLs for your sales team to prioritize!
- Sales-Assist Ready: given these leads are high customer fit but have not yet adopted your product effectively, you must nurture these accounts
- Not sure: given these leads have high product usage but low customer fit, you should analyze how these users engage with your product to determine if there is potential for new use cases or verticals
- Never touch: don’t spend your time on these leads
Developing your Product Qualified Lead Engine
How do the best Product-Led Sales experts build and iterate on PQLs? Last year we spoke to Aaron Geller who has done this 2x at DigitalOcean, Cypress and now again at QuickNode where he is VP of Sales and Customer Success.
Experiment first, then iterate
Aaron follows a simple formula for his PQL testing framework:
Step 1: Formulate a hypothesis for your PQL definition
- Try writing a mad-libs style statement: “We believe our best fit customer is ___________ because they use the product on average ____ days per ______ (week/month/year), for __________ use cases, their company profile matches ________ and they say the product adds _________ value to their company/workflow/workday.”
- Work backwards from the data and pick a key metric (i.e. usage spiked XX% in the last month)
Step 2: Pick a duration for the experiment
Don’t let your experiments run forever. Aaron recommends 30 to 60 days for an experiment, but this will also depend on your sales cycle.
Step 3: Define success metrics
You need clearly defined KPIs for any PQL experiment. Trying conversion or win rate is a good place to start, but don’t forget the softer metrics you may care about like overall efficiency of the process i.e. does the PQL make sales reps life easier?
Step 4: Pick your control and test groups (50/50 split or 75/25 - it's up to you)
This step is best done through a randomized process so you don’t create any bias in the groups.
Step 5: Analyze the results
Sometimes the results aren’t entirely black and white. According to Aaron you may need to talk to your sales team and get qualitative feedback in order to decide whether the PQL is worth it. The data doesn’t always have the answers so don’t be afraid to talk to your sales reps and then make a decision.
Key Takeaways on Experimentation:
#1 Be really clear on your KPIs for experiments
#2 Don’t be afraid to dig into inconclusive or “failed” experiments by talking to the team. You may not want to throw the baby out with the bath water necessarily
👉 Learn more activating your PQL hypothesis
PQL Ownership and Segmentation
When it comes to PQL ownership, PLG companies are still figuring it out, and many have different answers. What is clear is that it’s an effort that includes multiple teams to define and execute.
Who owns PQLs at PLG companies?
It’s a question we often ask PLS leaders during our PLS AMAs every month — and depending on the organization the answer is just a little bit different.
Although, from what we've seen at fast-growing PLG companies, not one department or person truly "owns" everything PQLs.
It's usually a mix of stakeholders taking care of different key functions.
For example...
- Product generates the PQL pipeline
- Growth defines & tests PQL hypotheses
- RevOps operationalizes PQLs
- Sales actions PQLs
Alexa Grabell, Pocus CEO, polled folks on LinkedIn recently to see what people think…
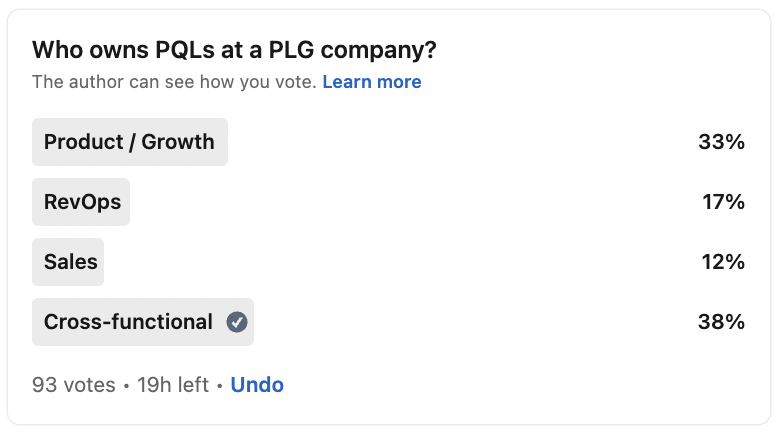
From the poll results and comments, it’s clear that the most important aspect is cross-functional communication and collaboration.
Defining a single PQL owner is more important, than who it is
Even though it’s a cross-functional effort, most orgs aim to define a single owner — a team to drive the strategy forward. It could be growth, a PM, or even a PQL project manager — although according to my LinkedIn poll growth/product take the lead.
This makes sense, while sales has a lot of domain expertise on what could be a PQL, ownership of the overall definition and data work is more aligned to growth teams. As with most things in PLS, it really depends on your GTM and how your teams are set up.
Bottom line advice: Pick a single owner to make sure the project doesn’t suffer from having “too many” cooks.
Does sales bias a PQL definition?
At first, I was pretty surprised sales seemed to be in last place when it comes to PQL ownership, after all, they are the ones who action them.
But, the truth is, even though PQLs are “for” sales, having the team be in charge of them could definitely bias PQL definitions towards feature usage sales knows will sell.
This is why doing both a qualitative brainstorm with your team AND a data driven analysis are both important.
Establishing ownership through PQL segmentation
Segmentation helps you define the who, what, and how: who you should target, what message you should use with the specific audience, and how the message will be communicated. Without a segmentation strategy, PQLs will become a free-for-all or, even worse, a vanity metric that sits on the proverbial shelf.
How you choose to segment PQLs will depend on how your team and GTM are set up. You might opt to segment based on the target market (eg. SMB, mid-market, enterprise). Or, you might choose to segment based on who owns the PQL (eg. sales team, sales-assist team. Or, maybe you’ll segment based on a playbook (eg. free-to-paid conversion).
Let’s dive into the last one, we’ve recently seen this strategy work well for teams who have robust sales and success teams that each have their own charters.
Product-Led Sales playbooks
Another way to segment PQLs is by aligning them to a playbook. We personally love using this strategy to identify your first set of PQLs at Pocus — oftentimes these playbooks naturally align to different teams and workflows that already exist in your GTM motion.
Here are some examples of PLS playbooks we’ve seen recently from Pocus customers:
- Enterprise consolidation
- Seat expansion
- Free trial conversion
- Startup program partners
- Free to enterprise conversion
Let’s take one of the above examples and define the PQL.
Example PLS playbook: Free trial conversion
Eligible customers: Signed up for a free trial, fits ideal customer profile, and significant usage in the product.
Example product usage signals:
- The account is NOT paid customer
- A user signed up for free trial
- User’s role is an ideal customer fit
- User’s industry is a target industry
- User invited 10+ teammates
- Account’s daily usage increased by 30%
Example owner: Account Executive
So, every time an opportunity hits a combination of the above signals, the free trial conversion PQL should be surfaced to the Account Executive. The AE will understand why they are getting this lead (to convert from free to paid), who the user is, and how the user has already engaged with the product to better inform their sales strategy.
Operationalizing PQLs
When you’ve got your PQLs defined and ready — the first thing we recommend you do is create a launch template.
Last year, we worked with Aaron Geller, PLS community ambassador, on a template for launching PQLs. He had devised a pretty easy to follow, but detailed process for operationalizing new PQLs in his previous roles. We’ve updated that template here. Use this as your project outline to help get everything set up.
Each section of the template informs a piece of the operationalization flow.
- Establish project details: Owner, team, roles, and launch date.
- Define your playbooks: Free trial, expansion, churn prevention.
- Define ops: Where are you pulling data from, where will you push data to, and how will you measure success.
Get your data infrastructure right
One of the biggest challenges of operationalizing your PQLs is in the data set up. Don’t set it up correctly and you might send messy data into your sales tools causing problems for your GTM team that will likely make them distrust that data all together.
Some tips for setting up your data stack:
- Before you dive into the data stack first identify what data you need (GTM team should do this), where it lives (Data team should do this), and flag if any data doesn’t exist.
- Invest in maintaining good data hygiene within your data warehouse and involve your data team in PQL discussions to make sure the data you need can be made available.
- Don’t push every data point to your CRM. Instead use scoring to push only specific PQLs to your CRM instead.
- Stitching together a PQL process across tools can get messy. Add a PLS platform like Pocus to help set up your playbooks, scoring models, and workflow for operationalizing data within each of your teams.
Make PQLs available to your team in their workflow
As discussed above, different PQLs should be surfaced to different team members based on the playbooks you are running. How should you surface these PQLs to your team so they actually get used? It depends on where your team likes to work.
You can push data to the CRM and set up lists or views that show all new PQLs. You can set up alerts in Slack for new PQLs and route them to a specific team channel or directly DM the owner of an account. Do this easily in a tool like Pocus or stitch this together manually in your CRM, Looker dashboards and workflow tools.
Train teams on how to follow up with PQLs
An often forgotten piece of the PQL puzzle is what to do once a PQL is surfaced. What channels should the GTM team use? What messaging? And at what cadence?
The golden rule for PQL outreach is PERSONALIZE. Use product usage data to inform your outreach instead of a generic message. Is this an expansion PQL at an account that has a ton of upside? Share a story of a similar customer who saw an increase in ROI when they added their entire team.

Surface, test, and action multiple PQLs with Pocus
{{form3a}}