Imagine the ability to harness all of the customer data that exists in your organization, run it through a magical machine learning model, and boom, you have answers to all of your burning questions. “Which accounts should my rep focus on?” “Where should marketing spend dollars?” “Which types of personas on the product lead to the best retention?”
The reality is we are still far away from making this happen with a snap of a finger, but ask any sales leader and this is their utopia. In the meantime, we’ve been working on ways to make this process feel as magical as possible within the realm of reality. We recently launched Predicts AI, a way for teams to easily experiment with AI-recommended Playbooks, the launch got a great response and sparked a lot of questions about the difference between Playbooks, traditional lead scoring, and AI-driven propensity models.
We’ll breakdown the 101 on:
- How Playbooks compare to scoring
- AI versus rules based methodologies and their trade offs
- How to choose which is best (scoring or Playbooks) based on your use case
Playbook vs. Scores
One of the most powerful levers in your go-to-market motion is the ability to prioritize where your team should focus their efforts. For years, lead prioritization stopped with the identification of marketing qualified leads (MQLs), where marketing teams would build scores to approximate the readiness of a buyer based on interaction with marketing campaigns. But MQLs only looked at top-of-funnel data with one goal, find warm leads that can be passed to sales for further qualification.
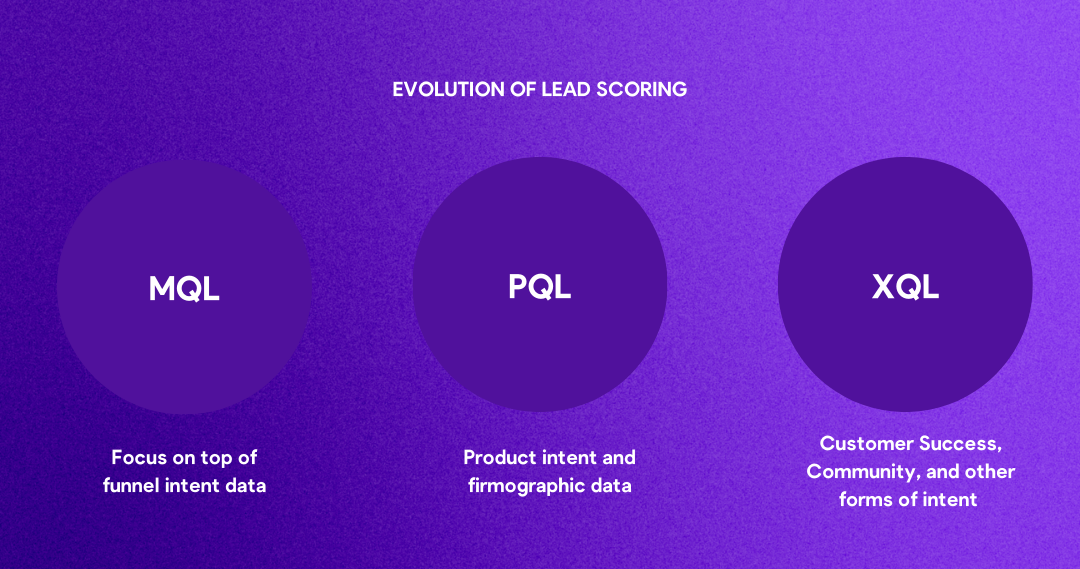
Then, with the rise of PLG companies in the last decade, we’ve seen focus turn to product-qualified leads (PQLs), where teams use product usage intent to score buyer readiness. More recently, we’ve seen this concept applied to post-sales with customer success qualified leads CSQLs and community qualified leads.
MQLs, PQLs, and CSQLs are all examples of traditional lead-scoring models used by go-to-market teams to prioritize their team's efforts.
A lead score is a composite of various signals that indicate a lead is promising. These signals can be all marketing intent data in the case of an MQL or based on product usage behavior, or a combination of product usage, customer fit, and marketing intent. The goal of a score is to make it easy for teams to sort a high volume of leads quickly.
Lead scores are not enough
Most lead scores are built as a single universal score that pinpoints a moment in time when a lead or account has the propensity to buy/convert. If you only have one goal, like converting free trial users to paid accounts, this score can be helpful.
But modern go-to-market motions are rarely that simple. PLG companies with self-serve products see more complex journeys from first use to paying customers to enterprise contracts.
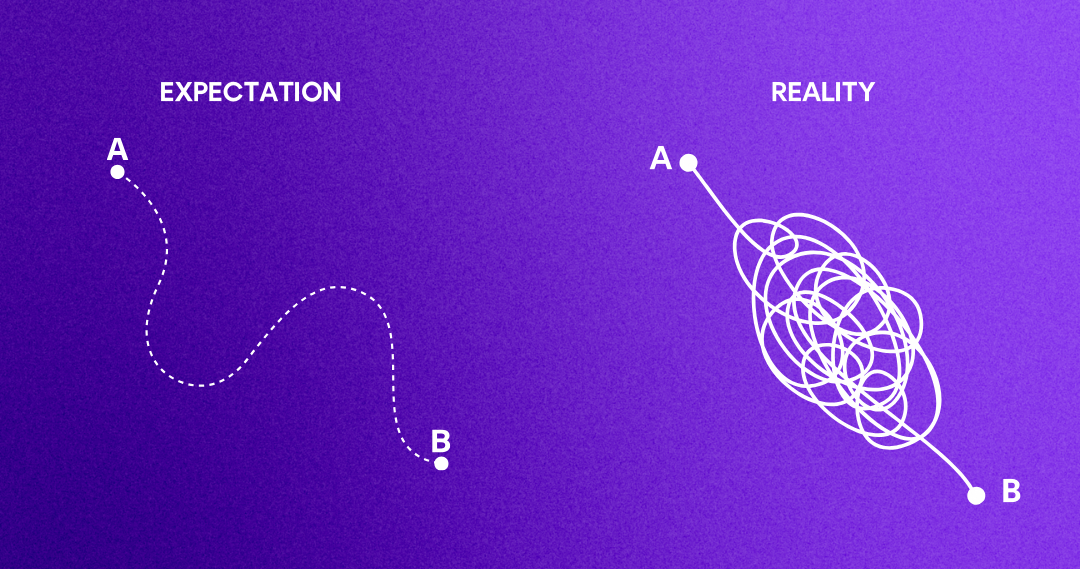
A PLG motion optimizes for an initial land first, leaving room for future expansion. If you apply a universal score to most PLG motions, you might over-index on the land and completely ignore the expansion motion (adding seats, upsells to higher-tiered plans, etc.)
If you have multiple goals across the customer journey, a single universal lead score won’t help you prioritize. A single lead score may not be enough for a few reasons:
- Doesn’t factor in the nonlinear nature of the buyer’s journey. There isn’t a single magical moment when a customer becomes product-qualified.
- Only looks at a single point in time on the customer journey instead of calculating a score at various stages.
- Built as a data science model that is not transparent to the go-to-market team making it difficult to trust fully.
- Scores alone don’t tell you what to do next or indicate the next best action.
Instead, PLG companies are rethinking prioritization and scoring in the context of goals along the entire customer journey - we call these Playbooks.
A Playbook is a prescriptive workflow that defines a specific goal for a cohort of customers and defines a set of plays your team should run to get closer to that goal. Instead of creating a score to help prioritize leads, define a goal and use Playbooks to test assumptions for which signals correlate most to conversion.
While scores look at many different attributes and compose them into an overall score, Playbooks are driven by specific attributes or signals. Playbooks are more effective in driving at a particular goal than a score. The specificity of why a lead or account qualifies for the Playbook helps your sales team understand how, when, and with what message to reach out. The granularity leads to better-timed and personalized outreach.
What about AI scoring?
With the hype around AI, AI-driven lead scoring has had a real resurgence. It makes sense, with all this data, surely you can put that into an AI model, churn out a score, and use that to automate your entire motion.
Traditional scoring, AI or not, will look at a point in time rather than consider the entire customer journey and all the goals that exist along this path. Especially in the Product-Led Sales context where the goals are increasingly complex (like going upmarket) and data volumes are lower, general AI lead scoring models will miss the nuances of the customer journey.
There are two primary considerations before using an AI scoring model - data volume and complexity of the goal.
- Amount of data volume (specifically conversion data) will directly impact accuracy. For example, most models require at least 50 conversions for statistically significant results. Do you have 50 conversions for enterprise upsells or seat expansions? Without data volume, scores won’t be effective for many goals.
- Complexity of the goal will impact how useful the score will be and how much context consumers of the score need. Who needs this score? Is it to power account-based ads on LinkedIn to drive free sign-ups within an account, or is it for sales to build the case for a cross-sell? If a goal is complex, you may need more context than a score can provide.

Let’s take moving upmarket as an example. AI scoring is not at all effective here. Traditional lead scoring doesn’t work when PLG companies move upmarket for a few reasons:
- The score is only as good as the data provided. If you are a PLG company that has mostly seen conversions, expansions and upsells from SMBs and mid-market accounts, then that lead score will tell you nothing about the new upmarket segment.
- Enterprise deals will fall through the cracks. The lead score will have you chasing what appear to be amazing opportunities that reflect your previous conversions while the new enterprise opportunities get ignored.
- Lead scores don’t have enough context. If you’re a PLG company moving upmarket, you cannot rely on a traditional lead score alone to shift from expanding SMB/mid-market deals to selling enterprise.
For these reasons, we believe AI models are more effective when used in the context of Playbooks. With Playbooks, the end goal is factored into the propensity of a particular signal. A signal like “director + user sign up” may have a higher likelihood of impacting your enterprise upsell goal versus a free-to-paid conversion.
Use Playbooks and Scores in your motion
You might be wondering if Playbooks are better suited for PLG companies and if traditional scoring might not be a fit. Do I need a lead or account score at all? In most cases, teams will use a Playbook and scores to operationalize their motion. Let’s take an example to illustrate how Playbooks and scores can work together.
Let’s say, as a go-to-market team, we are focused on two goals: 1) free trial conversion and 2) expansion. I am targeting slightly different segments of my user base with these goals: free trial users in the first case and existing paying customers in the second. The actions I want my team to take and when they take those actions will probably also differ. For this reason, we need to come up with distinct Playbooks that target these two different goals.
But, what’s common across both of these goals and customer segments? There is a baseline definition for our ideal customer profile (ICP) fit that should be considered, and product usage above a certain threshold. In this case, you can create a customer fit lead score and product engagement score to prioritize further leads that qualify for those goals.
Here’s an example of what this looks like in Pocus, this inbox shows leads that have qualified for a free-to-paid conversion Playbook and it’s sorted by a product engagement and customer fit score. This view helps reps quickly prioritize opportunities surfaced for a given Playbook.
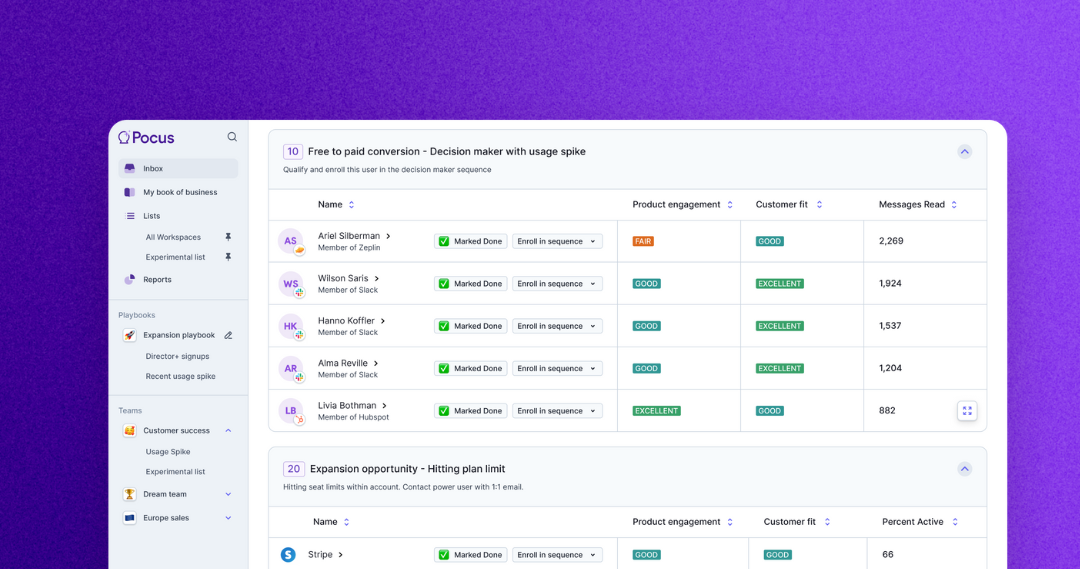
How to build your first Playbooks
Now that we understand the differences between Playbooks and Scores (and when to use them), what about AI? How does Predicts AI fit in?
Broadly, there are three options for how to get started building Playbooks, and the option or combination of options you choose will depend on your organization’s maturity, goals, and existing data available.
#1 Use your own strong hypotheses to create Playbooks
If your team has done the analysis - either quantitative (historical look at conversions) or qualitative (feedback from the field)- you might already have a strong hypothesis for the Playbooks you need to run to hit specific goals. In this case, the best way to determine if the Playbook is effective is to build and run.
The benefit of this approach is speed to get started and full control of the signals that power this Playbook.
#2 Start with proven templates based on PLS expertise
Do you have a strong hypothesis on Playbooks to run? If not, the Pocus team has you covered. We’ve spent hundreds of hours with the PLS community and customers to uncover patterns in how to build and test Playbooks. We’ll help you get started with some of the most commonly run Playbooks, customized to your specific needs.
Get the benefit of our library of Playbooks and PLS expertise combined with your expertise on customers and their buying behavior.
Need inspiration for Playbooks to build? Check out templates in our Playbook Library.
#3 Use Predicts AI to recommend Playbooks based on your data
If you’ve got a lot of historical data but not a great sense of where to begin, Predicts AI can recommend Playbooks with high propensity for conversion. What might typically require an internal data science project led by technical teams can be done without any code in Pocus.
Predicts AI looks at historical data for a particular goal, like free to paid conversion, and uses an AI model to pinpoint signals with the highest correlation to conversion. Use these recommended Playbooks alone or alongside your own hypothesis to test which Playbook is the best path to meeting your goal.
Often, a combination of all three approaches is required when experimenting with a Product-Led Sales motion. Playbooks allow you to test multiple hypotheses, whether driven by AI or your own analysis so you can double on what works with conviction.
Get started with Playbooks in Pocus
Need help building your paybooks? Get advice from our team of GTM experts.
{{widget-demo}}