This is part 1 of a 3 part series where we explore product-qualified leads (PQLs). We discuss what PQLs are, why you should be using PQLs, and how to define them to supercharge your sales team. The purpose of this guide is to help product-led growth (PLG) companies develop, establish, and operationalize their product qualified leads (PQL).
Read the entire series:
- Part 1: How to define and Identify Product-Qualified Leads
- Part 2: How to develop your Product-Qualified Lead Engine
- Part 3: Advanced PQL lead scoring concepts
What you'll learn:
- What is a product-qualified lead (PQL)?
- Pocus' framework for defining PQLs
- Building your PQL scenario matrix for customer segmentation
Is the MQL dead?
It’s nearing the end of the quarter and your sales leader comes knocking on your door and asks “can we have more Marketing Qualified Leads (MQLs) to fill my team’s pipeline?”...
You scramble to spin up new campaigns and refine your scoring to fill the funnel with more leads. The sales team complains that they can’t convert any of the leads to MQLs, that they’re “too cold”...
It’s no exaggeration - the world’s most successful SaaS go-to-market teams previously lived in this hellscape that none of us want to go back to - mindlessly chasing metrics like MQLs, filling sales pipelines with leads that have no hope of converting, and struggling to deliver actionable insights that could help close those bigger deals.
Enter product-led growth, a whole new world where your sales team doesn’t need to worry about chasing stale marketing leads. They can action something much better: Product Qualified Leads (PQLs).
What is a Product Qualified Lead (PQL)?
Unlike MQLs, which are based on the interactions a prospect has with your marketing content, PQLs are actual users of your product who have experienced your product’s core value. Not only that, but they also match your ideal customer profile, and have shown some purchase intent or buying signals.
Given PQLs fit your customer demographic and realized value out of your product, PQLs have a high likelihood of converting from their current subscription to a higher tier plan or enterprise expansion in a way that MQLs rarely do.
Why are PQLs important?
Why does defining, identifying, and nurturing PQLs matter?
Because, having the ability to identify the most promising sales opportunities, proactively reach the highest-value leads at the right time, increase conversion from free to paid to enterprise, and optimize internal sales processes all lead to the PLS holy grail:
- Improved customer experience
- Decreased customer acquisition cost (CAC)
- Accelerated time to revenue (velocity)
- Higher annual contract value (ACV)
Measuring fit, usage, and intent
A good PQL definition should capture signals from these three categories: (1) customer fit (2) product usage (3) and buying intent:
- Customer fit: how closely the account and user matches your Ideal Customer Profile (ICP)(eg. industry, geography, company size, user role/title)
- Product usage: account and user level behavior (eg. DAU, frequency, recency, time spent, free:paid ratio, feature usage, virality, invites sent)
- Buying intent: buying intent (visited pricing page, clicked "talk to sales", new seats added to account)
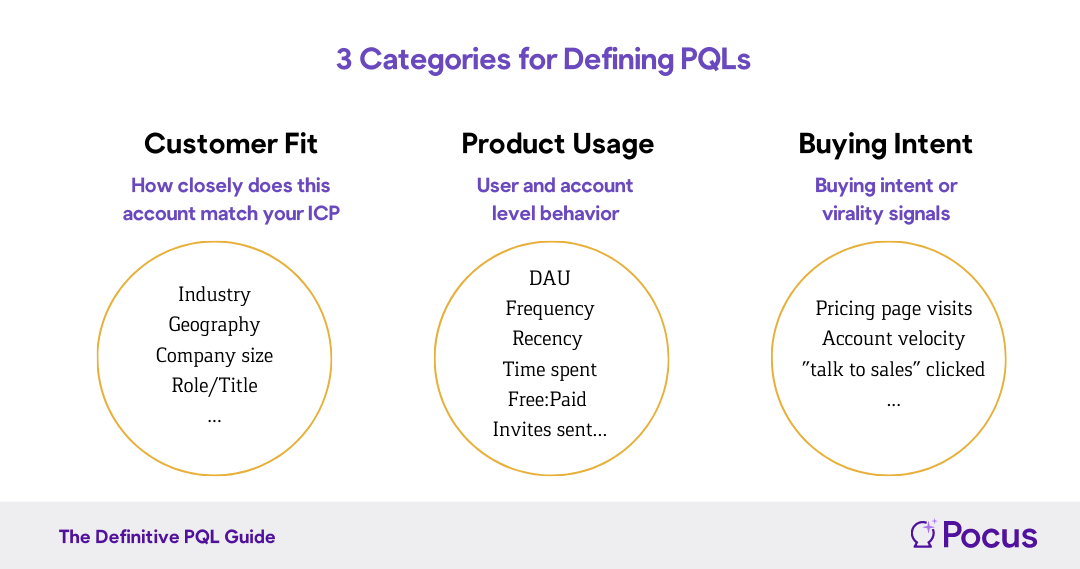
These categories help you decide how to identify and prioritize various scenarios of leads. You’ll ideally look for customers with great customer fit and high product usage / buying intent. But, you should also be aware of customers with great fit and low product usage, and vice-versa so that you so that you can nurture the account to ensure they are realizing value out of the product.
We find this matrix below helpful in understanding the various scenarios and mapping your PQL definition (hint: it’s up and to the right).
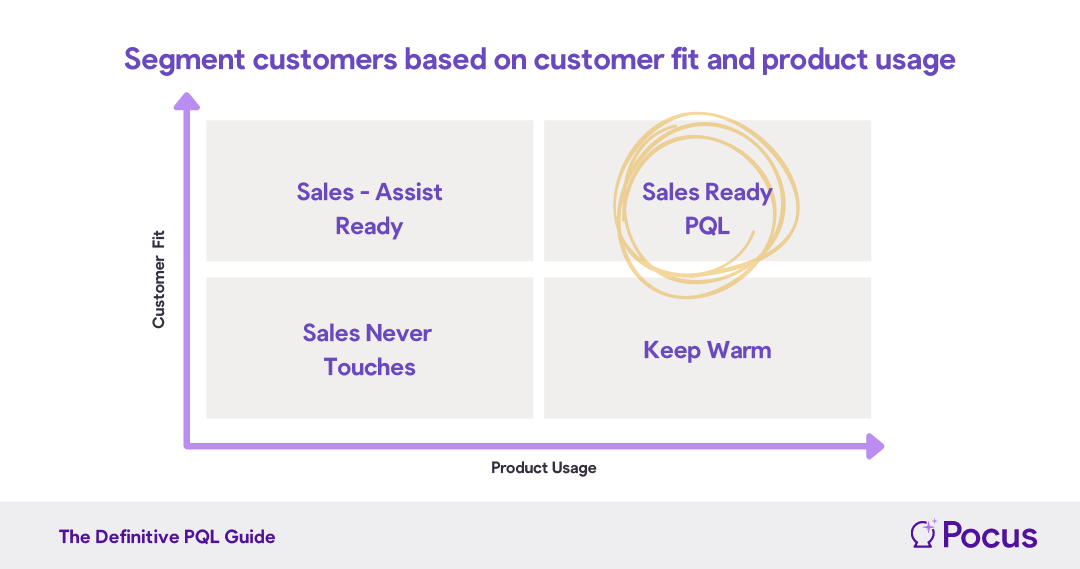
Each of the 4 segmentation scenarios require unique next steps from your sales team
- Sales Ready PQLs: these are your PQLs for your sales team to prioritize!
- Sales-Assist Ready: given these leads are high customer fit but have not yet adopted your product effectively, you must nurture these accounts
- Not sure: given these leads have high product usage but low customer fit, you should analyze how these users engage with your product to determine if there is potential for new use cases or verticals
- Never touch: don’t spend your time on these leads
We will dive deeper into operationalizing PQLs and determining next steps in part 2, but for now, let’s take a look at how you put leads into these categories.
How do you identify PQLs?
Before you define PQLs, it’s important to have a healthy self-serve flywheel up and running to fill the top of the funnel with potential leads. We’re not going to get into what it takes to build up your self-serve flywheel (that might have to wait for another post), but it’s important to understand why it’s necessary.
Setting up self-serve unlocks everything you need to successfully layer on sales.
With the self-serve user data, you’ll have an understanding of important signals like natural frequency of use, most heavily used features, and points of friction that ultimately help you understand who your best fit users are and how they behave in the product.
Once you have your self-serve flywheel up and running, it’s time to analyze some data..
5 Steps to help you identify PQLs
#1 Identify who would be a good customer (your ideal customer fit/profile)
This step should be familiar already. When you build a product for a particular market, you build it with some types of customers in mind, whether that’s a specific company size, industry, geography, or persona. This definition will continuously be refined and expanded after you bring your product to market and see what groups of users LOVE your product and those that only like your product.
Include the following in your ICP definition:
- Firmographic account data: geography, industry, company size, revenue, stage, etc.
- User data: role, title, willingness to adopt / try new products, seniority, etc.
- Revenue potential: willingness to spend, budget
If you started off with a broader definition of your ICP when your product first launched, it might be a good time to look at product data and validate that initial hypothesis or further refine. For example, your best fit customers may be in marketing broadly but you can further segment to identify specific marketing roles as best fit segments. Learning who uses your product, why they use it, and how, are all important contexts when considering account fit for PQLs.
#2 Understand how users get value from your product
A very important step in identifying PQLs is to identify signals that indicate a user has experienced your product's core value. In other words, your activation definition.
Your activation metric is defined as the moment a user meets a particular threshold of usage as mentioned above. You can look at metrics like frequency of usage overall, usage of a particular feature, recency of usage, free-to-paid ratio, etc.
A popular example of this is a slack workspace hitting 2000 messages sent. This is the primary indicator to the Slack team that a workspace is activated and ready to deepen their engagement.
#3 Identify purchase intent signals
Signals that tell you a user has some purchase intent are known as 🙋 hand-raisers.
These users are trying to tell you that they’d like to do more with your product. Some tell tale signs to pick out these users are if they ask questions about pricing, visit the pricing page to inquire, reach out to support for help, or hit a paywall within the product (usage limit or gated features).
The purchase intent is a key trigger for sales to step in and expand the engagement with the user.
#4 Formulate your PQL hypotheses
Most companies try to dive right into their PQL analysis, hiring tons of engineers and data scientists or outsourcing this to a consulting firm. This is not the answer. Get out a notebook and maybe an excel spreadsheet -- and that’s it.
You should start with a hypothesis about what your PQL is so that you can iterate over time. The truth is, most of the time your customer-facing team will have a strong (and nearly accurate) perspective of what the PQL is given they spend their day deeply understanding their customers.
So, through conversations with your sales, customer success, and product teams, identify hypotheses for what a “good” customer is for 1) customer firmographic data and 2) product usage data and 2) buying intent. Easy right?
Let’s dive into HOW you identify your initial hypotheses
- Talk to customer-facing teams and potentially end-users and find out what users they believe are highly engaged and what they know about those users.
- Work backwards from product data to discover cohorts of users that all love your product. Analyze customers that love your product and determine if those customers act in a certain way or use specific features.
- Map users to common company profiles / personas. Identify common patterns in company size, geography, business model, technographic profile that helps you group them into a particular firmographic profile
- Use 🔮 Pocus' mad-libs PQL statement:
Option 1:
“We believe our best fit customer is ___________ because they use the product on average ____ days per ______ (week/month/year), for __________ use cases, their company profile matches ________ and they say the product adds _________ value to their company/workflow/workday.”
Option 2:
“We believe our best fit customer account is [industry, geography, company size] with users that are [persona, title, seniority] and took [this action] in the product....”
You may also need to define more than one type of PQL.
For example, you may have multiple PQL funnels depending on your product and goals:
- Ready to expand an existing account
- Moving from the free tier to a paid plan
- Moving from subscription to enterprise licences
More on multiple PQL definitions and composite scores in part 3 of this series.
#5 Test and iterate your hypotheses in the real world
Now that you have a hypothesis for your PQLs, it’s time to test and iterate.
A) First, write down the objective behind testing this PQL and any relevant details of the PQL experiment - this can be in the form of a briefing document or create a section in your company wiki - just write it down.
B) Second, in this document you need to define how you will measure success.
Options include:
- Conversion from PQL:SQL
- Net Dollar Retention (NDR)
- Annual Contract Value (ACV)
- etc.
C) Third, pick your experiment parameters like…
- What is your A/B testing split? 50/50 or 70/30? Define who will be the control group and who will be in the testing group. It’s best to pick these groups in a randomized fashion.
- How long will the test run? 30 to 60 days is a safe bet but use your best judgement based on your products dynamics
D) Fourth, analyze the significance of the result.
- Was there a meaningful uptick in your chosen success metric?
- OR was it too close to call or inconclusive?
Remember that a failed test or inconclusive test may not mean that PQLs are not effective. Intead it may mean you need to tinker with your PQL definition, length of the test and try again. In the next part of this series we’ll hear from the team at Cypress and how they treat their experiments. (hint: not everything is so black and white).
Keep it simple...
PQLs can be a really powerful tool when making the switch from self-serve user growth to revenue growth via sales, but it can get complicated to operationalize and maintain within your team.
So what have we learned?
- Have a healthy self-serve flywheel and understanding of your activation definition before thinking about operationalizing PQLs
- A PQL must be an activated user (experienced value), fit your ICP (will turn into $$$), and has shown some signals or intent to purchase
- Always test & iterate your hypothesis before operationalizing
What’s next?
- How to operationalize your PQL process
- Assembling the right team, tools, and process for PQL success
If you made it all the way here, you’ll be excited to hear the next part of this series goes live next week. We’ll catch up with our friends at Cypress and Hightouch on how they ideated, tested, implemented and eventually automated PQLs in their sales process.